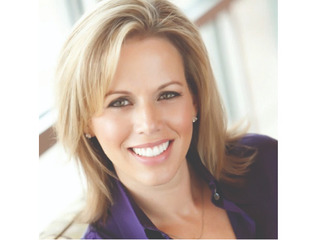
AI’s potential to be realized by a diversity of data pulled together in a centralized healthcare map
In my previous piece, I explored how big data improves patient outcomes, specifically by identifying useful datasets that lead to new insights about caregiving and treatments. This is only made possible thanks to artificial intelligence (AI), which enables new medical discoveries to emerge from the countless, seemingly indefinite combinations of data.
Without AI, the data trove, which is growing at a rate of 48 percent annually, would merely be a data dump. At the same time, without the data - the fundamental asset and foundation against which artificial intelligence is applied - AI’s full potential will never be fully realized.
AI is real. AI isn’t just software that has perfected pattern matching, but software that imagines, deduces and infers. This type of software is in its infancy as it’s estimated that only $500 million in revenue was generated from healthcare AI last year -- a small sum compared to the $100 billion healthcare IT market.
That said, healthcare AI is expected to quickly grow to $8.6 billion by 2025. A decade from now, it’s difficult to predict how AI will have advanced screenings, diagnostics, treatments and ultimately patient outcomes. The possibilities are endless, which is a big part of what makes this moment so exciting.
In this piece, we’ll examine the evolution of AI in healthcare; how we’re thinking about AI at Komodo Health and the importance of robust, diverse data streams (looking at both breadth and depth of data); and how others collectively driving this movement forward are experiencing success and identifying emerging challenges.
The building blocks of AI
In the last two years, the buzz around AI has dramatically accelerated, particularly in cases such as when doctors in Beijing competed against, and lost to, A.I. computers, which were able to better recognize illnesses on magnetic resonance images of a human brain.
In healthcare, AI has required an asset that heretofore did not substantially exist until recently - big data.
“Before the AI buzzword was the ‘big data’ buzzword, a time when we were helping health systems simply collect and store the data,” said Chris Gough, Worldwide General Manager of the Health and Life Sciences Group at Intel. “We did a lot of work around building data lakes and natural language processing which were good stepping stones to get us ready for AI, so that we could eventually implement AI capabilities to leverage the data.”
For Dr. Tina Moen, PharmD, Senior Deputy Chief Health Officer and Chief Pharmacy Officer at IBM Watson Health, AI has already had an impact but it continues to quickly evolve with the diversity of perspectives and new data.
“Fantastic progress has been made with AI,” she said, referring to the Mayo Clinic’s use of Watson to connect patients to critical clinical trial options. “But we’re still at the very, very beginning. There’s still so much to come, and that comes through continued partnerships and an “it-takes-a-village” mentality to acquire and link robust datasets and a diversity of talents and perspectives to make progress.”
In order to realize AI’s true potential in healthcare, we need to work off of the largest and most complete set of data available at any given moment. At Komodo Health, we believe the best machine learning models can only be developed and deployed when trained on the best data.
Diversity of data leads to precision medicine and invention
The beauty of AI is its ability to take data from multiple sources and surface new insights with limited to no human intervention required. In Plato’s cave allegory, men perceived the world as shadows on the cave wall because they had a limited view, or limited data, of reality. In a similar vein, the formation of new datasets is now painting an alternate truth or one that is more precise and accurate than what we saw before.
“If you think about AI in the context of what it really means for precision medicine, we’re only starting to scratch the surface. AI makes true precision health possible when you think about it in the context of being more than just how genomics impacts someone’s disease,” said Intel’s Gough. “It’s more than just genomic data, but combining it with clinical data, claims data, medical images, and real-time data generated from sensors that patients wear or environmental information around the patient, then using AI capabilities to determine the right intervention, for the right person at the right time.”
IBM’s Moen concurs. “We’re having conversations around weather data and how we can apply that to what we know about health and the impact that weather has on health. We’re also looking at social determinants, e.g., your zip code, your income level, etc.. Health is impacted by so many things and we have to ask ourselves: What are the different types of data? What do we have? What can we get access to? What don’t we know and still need to get?”
Fran Ayalasomayajula, Head of Population Health Portfolio, Worldwide Healthcare at HP, reiterated the same point, noting that there’s immense amounts of data generated outside of the clinical setting that represent a big opportunity for artificial intelligence.
“In healthcare, we tend to focus on the one percent, or the data that resides from the interactions that patients have had within the clinical setting,” she said. “Where we have an opportunity is the 99 percent which is where people live, work, play and pray. That’s all the data that exists outside of that environment. We are looking to leverage that in different ways.”
To this end, HP’s focus is on building the hardware that can capture data that we haven’t even thought of.
“It’s really about the design of the technology and the design of the hardware devices that can capture all sorts of other data,” she said, adding that it’s optimally used when there’s AI that can take advantage of such technology as holographic imaging to create virtual scenarios and virtual adaptations for addressing the challenges of, for example, autism. “Those are challenges that we have and that we face because our hardware innovations require the collection of a lot of data, some of which doesn’t exist today.”
Centralized healthcare map to optimize AI
The theme, if you haven’t noticed, is that each new dataset applied or layered over existing data oftentimes leads to different discoveries and insights. The challenge is bringing all the new datasets together in a format against which we can apply machine intelligence. The challenge is having some semblance of that first layer already in place and ensuring it’s accessible.
“One of the hurdles, and to some degree it’s a technological one, is access to the data. Particularly here in the United States, it’s very hard for individual patients to access their medical records,” said Intel’s Gough. “There’s been lots of activity in the past year both by CMS and HHS to help patients get more access to their data, so we’re moving in the right direction toward making electronic health record environments more open.”
Gough also observed that there’s certain centralization of data happening, but more needs to be done.
“There is a lot of siloed data in Healthcare. It’s challenging to exchange and share data across different Health-IT systems and vendors, and challenging to store the multi-modal data types described above in a way that enables modern techniques like predictive analytics and deep learning. Data lakes with integrated data management and governance capabilities and interoperability initiatives like SMART on FHIR are promising steps, but more work needs to be done. What may still be missing is a core platform build for health analytics from the ground up.”
At Komodo Health, we realized that the core platform of healthcare data had to do two things: 1) it had to be flexible and extensible enough to allow additional data sources to be layered on in the future, and 2) it needed to do that in a way that always preserved the privacy of the underlying patient data.
Conclusion
In order to fully appreciate the progress of AI in healthcare, we need to understand the big picture. While the work of laying the foundation may not always be visible, it’s imperative we get that right. There are no constraints around the core technology, and progress continues to be made.
“Our partners and experts in applying AI techniques to different problems have a great sense of what is available and a great sense of the challenges of data. They know the problem, which is the first challenge, and have many techniques to address those problems -- the data cleanliness or data scarcity or too much data,” said IBM’s Moen. “AI will continue to evolve. In five years, we’ll look back and say, ‘I can’t believe we were doing it like that in 2019,’ but that’s an evolution as opposed to a gap.”
AI’s ability to transform healthcare is unprecedented with regards to the advances we will see in diagnostics, screenings, preventions, treatments and cures. It’s an exciting time for us, for the healthcare industry overall, and for the companies ushering in a new era of change and innovation.
(Image source: pulse.embs.org)
Related Companies, Investors, and Entrepreneurs
Komodo Health
Startup/Business
Joined Vator on
The most powerful insights come from the most comprehensive data. Our Healthcare Map empowers clients and partners to achieve optimal, cost effective outcomes for every patient in the US.

Fran Ayalasomayajula
Joined Vator on
Population Health Technologies and Innovations Lead, Worldwide Healthcare, HPRelated News
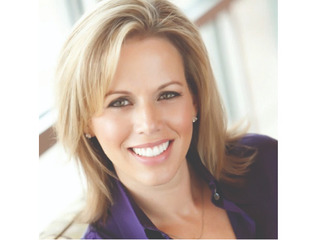
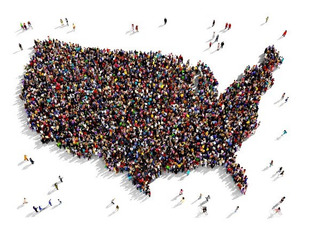
Will one company make sense of health data?

How big data is improving patient outcomes
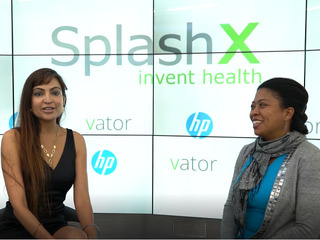